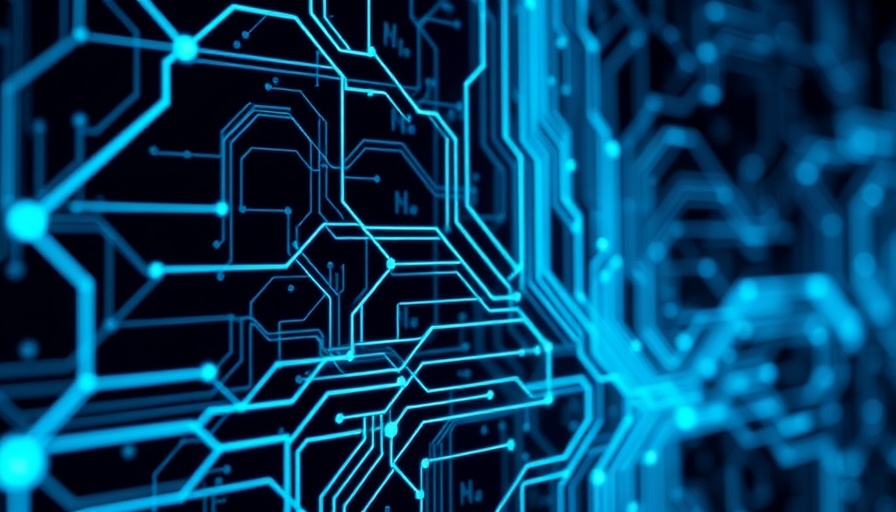
Understanding Synthetic Data's Importance in AI Learning
Synthetic data has emerged as a cornerstone resource for advancing AI learning and machine learning models. Unlike traditional datasets, synthetic data allows for expansive analysis while protecting individual privacy. Imagine being able to train AI for sensitive applications like healthcare without exposing real patient records. This advancement is paving the way for a more robust AI ecosystem.
Navigating the Complexities of Good Synthetic Data
Yet, not all synthetic data is created equal. Its quality is contingent upon its intended use, or “fit for purpose.” This means analytics practitioners must strike a balance between the similarity of synthetic datasets to actual data and the level of privacy required to protect sensitive information. For instance, when modeling customer behavior, high similarity might be desired; however, over-reliance on real data patterns can risk compromising privacy. To mitigate this, strategies such as differential privacy can be employed, introducing necessary differences to obscure sensitive patterns.
A Comprehensive Checklist for Assessing Synthetic Data
With the significance of evaluating synthetic data established, here’s a practical checklist:
- 1. Check for Bias Amplification: Synthetic data can inadvertently perpetuate or even amplify biases found in the original datasets. It is critical to analyze both original and synthetic data for biases to ensure fairness in outcomes, especially in critical fields like healthcare.
- 2. Balance Share of Data Segments: Certain synthetic datasets can bolster underrepresented segments, which is especially useful in fraud detection or healthcare studies where certain demographics may lack sufficient samples.
- 3. Privacy and Security Measures: While synthetic data can enhance privacy, practitioners must also ensure that no inadvertent disclosures occur. Techniques such as differential privacy help secure data while deploying security measures that keep synthetic datasets safe from breaches.
The Future of AI Learning with Synthetic Data
Looking ahead, the evolution of synthetic data generation tools will revolutionize how we approach AI science. With increasing data regulations and privacy concerns, understanding and implementing robust synthetic data practices will be essential for responsible AI learning paths. Companies will need to prioritize developing technologies that incorporate these best practices to stay ahead in the competitive landscape.
Why Everyone Should Care About Synthetic Data
Synthetic data isn’t just a technical detail for data scientists; it has broader implications for society. As AI-driven decision-making becomes more prevalent, ensuring fairness, diversity, and privacy in training datasets is critical. This security will enhance trust in AI applications across various sectors, from finance to healthcare.
Final Thoughts and Call to Action
For those looking to delve into the realms of AI learning, understanding synthetic data is paramount. By harnessing the insights presented in this checklist, readers can contribute to the advancement of more responsible AI frameworks that prioritize privacy without sacrificing performance. Now is the time to learn more about how these innovative data strategies can shape the future of technology and society’s relationship with it.
Write A Comment