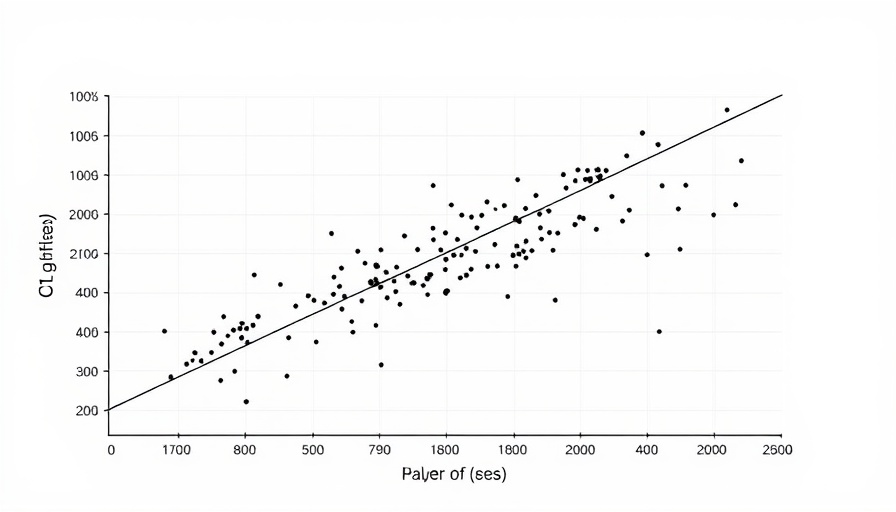
Understanding George Box's Wisdom on Model Assumptions
In the realm of statistics and data science, one quote resonates deeply: "All models are wrong, but some are useful." Attributed to the esteemed statistician George Box, this aphorism succinctly captures the essence of how we engage with models in our quest for understanding complex phenomena. However, when looking into the origins of this statement, it becomes clear that there is a rich history woven through Box's work that offers invaluable insights for today's data-driven landscape.
Modeling: A Necessary Imperfection
George Box coined the phrase in his 1976 article, "Science and Statistics," where he articulated the iterative nature of modeling. He suggested that while models can never capture the full reality, they still serve a fundamental role in informing our understanding. Box notes, “Since all models are wrong... the scientist must be alert to what is importantly wrong,” reinforcing the idea that the utility of a model lies not in its correctness, but in its applicability and the insights it yields.
The Duality of Models: Insights vs. Accuracy
The beauty of Box's assertion lies in its duality: all models simplify reality, yet within these simplifications, we can discover trends and make predictions. This is particularly relevant in AI learning. Models in AI often undergo extensive training, and while they aim for accuracy, understanding their limitations allows developers and users to harness their capabilities effectively. For instance, in AI science, algorithms can generate predictions based on datasets, providing insights even if they do not account for every variable or nuance of real-world scenarios.
Applying Box's Insight in Today’s AI and Machine Learning Realm
In the rapidly evolving fields of artificial intelligence and machine learning, the principles that Box outlined are more pertinent than ever. As businesses integrate AI technologies, it is crucial to acknowledge that these systems operate on models that may not always reflect accurate real-world complexities. Box’s perspective encourages practitioners to focus on the question: "How might these models serve useful purposes despite their flaws?" This insight is key as organizations design AI learning paths that guide users in leveraging models for tangible benefits.
Example: The AI Bias Problem
A pertinent illustration of Box's aphorism can be seen in discussions around algorithmic bias. To ensure fairness in AI systems, developers must recognize that the models they create are based on historical data, which may inherently carry biases. Understanding that all models are wrong—in the sense that no model can account for every factor—opens the floor to creative solutions and discussions around bias mitigation strategies. Stakeholders can ensure that models serve their intended purposes, while continuously refining them with new data and insights.
Conclusion: The Pragmatic Approach to Modeling in AI
As we advance towards greater sophistication in AI technology, embracing the notion proposed by Box can enhance our approach to model building. Instead of seeking the elusive 'correct' model, we can focus on developing models that provide valuable approximations of what we seek to understand and predict. This pragmatic approach not only aids in decision-making processes but also cultivates a deeper appreciation for the models we engage with in AI science.
Reflecting on George Box's insights equips us for a future where models will continue to play a critical role in navigating complexities across various domains. Understanding their limitations—yet still actively utilizing them—can lead to substantial advancements in AI learning and broader technological applications.
Write A Comment