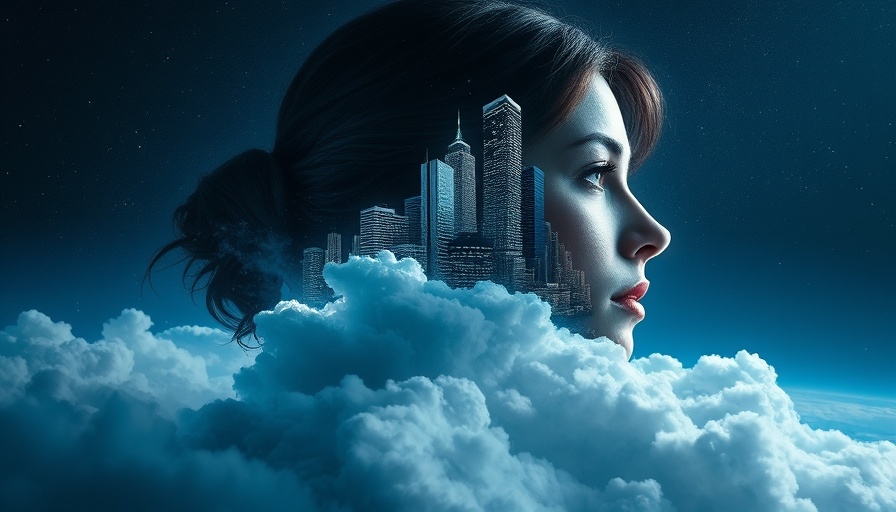
Understanding the Next Generation of AI Reasoning
Large Language Models (LLMs) have evolved from their early functionality, which primarily revolved around text completion, into sophisticated systems capable of more complex reasoning tasks. This progression marks a significant shift towards true artificial intelligence that can solve problems, analyze code, and even learn from its mistakes. But the question remains: how are these models improving their reasoning capabilities?
The advancements are largely thanks to several innovative techniques that encourage LLMs to think and reason more like humans. This article delves into five of the most important methods transforming reasoning within LLMs.
1. Chain-of-Thought Prompting: Encouraging Step-by-Step Reasoning
One of the earliest improvements in enhancing LLM reasoning is the Chain-of-Thought (CoT) prompting method. Rather than merely providing a direct answer, this approach guides the model to articulate its thought process, enabling it to break down complex problems into smaller, manageable steps. For instance, asking “What’s 17 times 24?” can become “Let’s think step by step,” prompting the model to explain the multiplication in parts.
This technique, first formalized in 2022, has laid the groundwork for enhancing the reasoning abilities of models like OpenAI's o1 and its successor o3, the latter being designed to emulate a thought process by pausing to reflect and refine answers. Such methodologies help prevent models from jumping directly to conclusions and better manage intricate logic tasks.
2. Inference-Time Compute Scaling: More Resources for Tough Questions
Humans typically allocate more time to complex questions, and LLMs can now mimic this behavior through Inference-Time Compute Scaling. This technique increases the computational resources allocated during response generation, allowing models to explore multiple reasoning paths before determining the best answer. For instance, OpenAI’s o3-mini offers different levels of reasoning effort, demonstrating significant improvements in challenging tasks like mathematics and coding.
A related optimization strategy is budget forcing—through which models use specific tokens to control reasoning depth. By guiding the models with tokens suggesting pauses and self-verification, the results are longer and more accurate responses without necessitating modifications to the model's underlying weights.
3. Reinforcement Learning: Rewarding Logical Outcomes
Training models to reason better can also involve introducing reinforcement learning (RL)—where models receive rewards for producing correct logical conclusions. This methodology was pivotal in the development of models like DeepSeek-R1, which trained mainly on RL principles, leading to the emergence of robust reasoning capabilities. However, initial iterations faced issues like instability. Consequently, final iterations have integrated multi-stage training, combining RL with supervised techniques for enhanced readability and reliability.
4. The Power of Enhanced Contextual Understanding
A successful AI marketing agency knows that understanding context is paramount. The LLMs now leverage vast amounts of data to provide contextual insights, which is crucial for developing personalized marketing strategies. By grasping the intent behind queries and tailoring responses based on user context, LLMs can serve as indispensable tools in digital marketing services.
5. Emerging Techniques and Future Predictions for AI
The capabilities of LLMs are only set to increase as further research and optimization develop. Techniques such as budget forcing and simulated reasoning will likely see broader applications, allowing businesses to leverage advanced AI tools effectively for various needs.
The integration of newly developed techniques into practical applications heralds a new era for AI, particularly for entrepreneurs seeking to capitalize on AI-driven marketing strategies. As these models evolve, they promise to unlock new business software solutions and innovative marketing methods tailored to individual customer needs.
Conclusion: Embracing the AI Revolution in Marketing
As artificial intelligence continues to advance, understanding and utilizing these new reasoning methods can provide businesses with a competitive advantage. AI applications in marketing are burgeoning, ensuring personalized experiences for consumers while optimizing operational efficiency. Businesses looking to integrate AI capabilities should reach out and explore what tailored solutions could enhance their strategies. Contact Us For Your AI Needs.
Write A Comment